Levels of autonomous driving represent a spectrum of vehicle capabilities, ranging from driver-assisted systems to fully automated operation. This exploration delves into the nuances of each level, from basic driver aids to sophisticated self-driving features, highlighting the evolving technological landscape.
Understanding the different levels of autonomy is crucial for comprehending the current state and future potential of this transformative technology. Each level presents distinct technological components, safety considerations, and societal implications. From the basic sensor systems to the sophisticated algorithms, the evolution of autonomous vehicles is rapidly reshaping the transportation landscape.
Defining Autonomous Driving Levels
Autonomous driving, a rapidly evolving technology, is categorized into distinct levels of automation. Understanding these levels is crucial for comprehending the capabilities and limitations of current and future vehicles. This classification system helps consumers make informed decisions and promotes responsible adoption of the technology.These levels define the extent of automation in a vehicle’s driving functions. Each level represents a progressively higher degree of autonomy, with increasing capabilities but also varying degrees of human oversight and control.
The transition from one level to another is marked by a shift in the responsibilities between the driver and the vehicle’s automated systems.
Autonomous Driving Levels Overview, Levels of autonomous driving
The levels of autonomous driving, ranging from Level 0 to Level 5, represent a spectrum of automation capabilities. They clearly define the extent of vehicle automation and the required driver intervention at each stage.
- Level 0: No Automation. This level represents vehicles without any automated driving features. The driver is entirely responsible for all aspects of driving, including steering, acceleration, braking, and lane keeping. Examples include all traditional cars without advanced driver-assistance systems (ADAS).
- Level 1: Driver Assistance. Level 1 vehicles incorporate automated features for specific tasks. Examples include adaptive cruise control and lane-keeping assist. The driver retains primary control over the vehicle’s operation. These features provide limited assistance but do not take over complete control of the vehicle’s operation.
- Level 2: Partial Automation. Level 2 vehicles can automate two or more driving tasks simultaneously, such as steering and acceleration. However, the driver must remain attentive and ready to take over control at any time. Examples include some advanced cruise control systems, adaptive cruise control, and lane-keeping systems.
- Level 3: Conditional Automation. In Level 3, the vehicle can manage most driving functions under specific conditions. The driver is still required to be ready to take over, but the vehicle can handle the tasks automatically under appropriate circumstances, like highway driving. Examples are still limited, and the technology is evolving.
- Level 4: High Automation. Level 4 vehicles can perform most driving tasks in various situations without human intervention. The vehicle can handle the complexities of driving in various situations, such as urban environments. However, the driver still needs to be prepared to take control if the vehicle encounters an unforeseen circumstance. Examples are still limited but are being actively developed by manufacturers.
- Level 5: Full Automation. This represents fully autonomous vehicles. These vehicles can perform all driving tasks in any situation without any driver intervention. They can handle all driving scenarios, including complex urban settings and unexpected situations. Examples of Level 5 vehicles are still largely theoretical and under development.
Key Differences Between Levels
The key differentiator between autonomous driving levels is the degree of human intervention required. Level 0 requires complete human control, while Level 5 removes all human intervention. The transition between levels reflects a gradual shift in responsibility from the driver to the vehicle’s automated systems.
Comparison Table
The table below summarizes the levels of autonomy, capabilities, and typical use cases for each level.
Level | Autonomy | Capabilities | Typical Use Cases |
---|---|---|---|
0 | No Automation | Driver performs all driving tasks | Standard vehicles |
1 | Driver Assistance | Limited automated functions (e.g., cruise control) | Highway driving, some city streets |
2 | Partial Automation | Simultaneous control of steering and acceleration | Highway driving, some city streets |
3 | Conditional Automation | Vehicle manages most driving functions in specific conditions | Highway driving, some city streets, limited situations |
4 | High Automation | Vehicle manages most driving tasks in various situations | City driving, specific routes, certain conditions |
5 | Full Automation | Vehicle manages all driving tasks in any situation | Various environments, long-distance travel, specific situations |
Technological Components of Each Level
The advancement of autonomous driving hinges on a sophisticated interplay of technological components. Each level of autonomy relies on specific sensor technologies, sophisticated software, and cutting-edge artificial intelligence (AI) algorithms. Understanding these components reveals the intricate engineering behind self-driving vehicles and illuminates the pathways to future capabilities.
Sensor Technologies
Sensor technology forms the foundation of autonomous driving systems. Different levels demand varying degrees of sensor accuracy and reliability. The precise data captured by sensors enables the vehicle to perceive its environment, enabling crucial decision-making. The accuracy and range of these sensors directly affect the vehicle’s ability to operate safely and reliably in diverse conditions.
- Cameras are crucial for visual perception. They capture images of the surrounding environment, enabling object detection, lane marking recognition, and traffic signal identification. Modern cameras utilize advanced image processing algorithms to extract meaningful information from raw video data. For example, specialized algorithms can differentiate between a pedestrian and a parked car, enabling safer navigation.
- Radar provides information about the distance and speed of objects. Radar sensors are especially valuable for detecting objects in adverse weather conditions, such as fog or rain, where cameras may struggle. By measuring the time it takes for radio waves to bounce off objects, radar systems can precisely gauge their position and velocity. This data is instrumental in maintaining safe distances from other vehicles and obstacles.
- LiDAR (Light Detection and Ranging) utilizes lasers to create detailed 3D maps of the surroundings. LiDAR sensors provide highly accurate measurements of the distance and shape of objects, which is vital for complex maneuvers and navigation in cluttered environments. The high resolution and accuracy of LiDAR data are especially important for Level 4 and 5 autonomous vehicles operating in challenging situations, such as navigating narrow streets or parking in tight spaces.
Software and Algorithms
The software and algorithms are the “brains” of the autonomous vehicle. They process the sensor data, enabling the vehicle to perceive its environment, make decisions, and execute actions. The sophistication of these algorithms is crucial for achieving higher levels of autonomy.
- Perception software uses the data from cameras, radar, and LiDAR to create a comprehensive understanding of the environment. This involves identifying objects, estimating their position and velocity, and classifying them (e.g., pedestrians, vehicles, traffic signals). Advanced machine learning algorithms are increasingly used for object recognition, improving accuracy and robustness in various conditions.
- Decision-making algorithms determine appropriate actions based on the perceived environment. These algorithms consider traffic rules, potential hazards, and the vehicle’s intended path. Sophisticated algorithms weigh different scenarios, selecting the safest and most efficient course of action. Real-time decision-making is critical for safe and responsive driving.
- Control algorithms translate the decisions into actions. These algorithms control the vehicle’s steering, acceleration, and braking systems to execute the desired maneuvers. Advanced control algorithms are essential for maintaining stability and precision, particularly in dynamic situations. For instance, algorithms need to precisely calculate braking and acceleration to smoothly navigate turns.
Advancements in AI and Machine Learning
Artificial intelligence and machine learning are instrumental in enhancing the capabilities of autonomous driving systems. By enabling the systems to learn from vast amounts of data, AI and machine learning lead to significant improvements in accuracy, reliability, and adaptability.
- Machine learning algorithms are trained on massive datasets of sensor data to improve object recognition and environment understanding. This learning process enables the algorithms to adapt to various conditions, such as different weather patterns or traffic densities.
- Deep learning techniques, a subset of machine learning, have proven exceptionally valuable in autonomous driving. Deep learning models excel at recognizing complex patterns in sensor data, enabling more accurate and reliable perception. This is especially crucial in scenarios with diverse and dynamic situations.
- Reinforcement learning allows the autonomous vehicle to learn through trial and error. The vehicle receives rewards for safe and successful actions and penalties for mistakes. This learning process enables the vehicle to adapt to unforeseen circumstances and optimize its behavior over time.
Integration of Technologies
The different technologies work together seamlessly to enable various levels of autonomy. The combined capabilities of sensors, software, and AI enable the vehicle to perceive its environment, make informed decisions, and execute actions autonomously. The interplay of these components defines the level of autonomy achieved.
Sensor Type | Function | Relevance to Autonomous Driving Levels |
---|---|---|
Cameras | Visual perception | Essential for all levels, particularly for object recognition and lane detection. |
Radar | Distance and speed measurement | Crucial for obstacle avoidance and safe distance maintenance, especially in challenging weather conditions. |
LiDAR | 3D mapping of the environment | Critical for high-level autonomy, enabling accurate perception in complex situations and dense environments. |
Safety Considerations and Regulations
Autonomous vehicles, while promising, present unique safety challenges. Ensuring the safe operation of these vehicles requires robust safety protocols and comprehensive regulations, adapted to the evolving levels of autonomy. The complexity of the technology and the potential for human error, particularly at lower levels, necessitate a layered approach to safety.The development and implementation of safety features are intrinsically linked to the level of autonomy.
Basic safety systems, like airbags and anti-lock brakes, are crucial at all levels. However, the sophistication of the safety features must increase as the vehicle gains more autonomy, requiring more intricate sensors, advanced algorithms, and redundancy. The introduction of these advanced systems demands careful evaluation and scrutiny, considering potential vulnerabilities and ethical implications.
Safety Features Implemented at Each Level
A comprehensive suite of safety features is essential at each level of autonomous driving. These features range from basic driver-assistance systems to sophisticated algorithms for decision-making in complex scenarios.
- Level 0 (No Automation): Safety relies entirely on the human driver. Basic safety features, such as airbags, anti-lock brakes, and electronic stability control, are the primary safeguards. These features are designed to mitigate risks associated with human error and unexpected situations.
- Level 1 (Driver Assistance): Driver assistance systems, like adaptive cruise control and lane departure warning, are incorporated. These features enhance safety by providing alerts and automated support in specific situations. Safety relies heavily on the driver’s vigilance and ability to respond to the assistance offered by the vehicle.
- Level 2 (Partial Automation): Vehicles at this level can perform tasks like steering and accelerating in certain conditions. Safety systems often include enhanced driver monitoring and automated emergency braking. The systems need to be designed to handle potential malfunctions and to revert control to the human driver quickly and safely.
- Level 3 (Conditional Automation): The vehicle can handle a wider range of driving tasks, but the driver must be prepared to take control under certain circumstances. Advanced driver-assistance systems, robust monitoring systems, and automatic intervention protocols are critical. Redundancy in systems and fail-safe mechanisms are crucial.
- Level 4 (High Automation): The vehicle is capable of handling most driving tasks under normal conditions. Extensive sensor integration, advanced algorithms, and redundancy are necessary to ensure safety. Safety systems focus on handling diverse driving situations and ensuring the vehicle can react appropriately to unexpected events.
- Level 5 (Full Automation): The vehicle handles all driving tasks without human intervention. Highly advanced sensor technology, fault-tolerant algorithms, and extensive simulations are critical to ensuring complete safety. Safety relies on the vehicle’s ability to anticipate and react to various scenarios effectively.
Safety Standards and Regulations Governing Autonomous Vehicles
Globally, various safety standards and regulations are being developed and implemented for autonomous vehicles. These regulations are intended to ensure that autonomous vehicles operate safely and predictably. The standards vary based on jurisdiction and the level of autonomy.
- International Standards: Organizations like the International Organization for Standardization (ISO) are working on developing standards for autonomous vehicles. These standards will cover various aspects, including testing procedures, safety protocols, and data management. International harmonization is crucial to promote the safe deployment of autonomous vehicles globally.
- National Regulations: Many countries are developing specific regulations for autonomous vehicles. These regulations typically address the testing, deployment, and operation of autonomous vehicles within their respective jurisdictions. Differences in regulatory frameworks across countries could create challenges for the global rollout of autonomous vehicles.
Safety Incidents and Near Misses
Incidents and near-misses involving autonomous vehicles are a key area of ongoing study and development. These incidents often highlight the need for further improvements in safety features and regulatory frameworks.
- Level 2 Incidents: Some incidents involving Level 2 vehicles highlight the importance of driver vigilance and the need for clear communication between the driver and the vehicle’s automated systems. The need for the driver to remain engaged in the driving task remains paramount.
- Level 3 Incidents: Issues with the transition of control from human to vehicle systems are often reported in Level 3 incidents. The reliability and responsiveness of these systems are vital to maintaining safety.
Ethical Considerations in Autonomous Driving
Ethical dilemmas are inherent in autonomous driving. Algorithms must be designed to make decisions in complex situations where there are no perfect answers. Ethical frameworks for autonomous vehicles are being developed to ensure these decisions are made in a responsible manner.
- Moral Dilemmas: Autonomous vehicles face ethical dilemmas, such as deciding between the safety of passengers and pedestrians in unavoidable accidents. The programming of these vehicles requires careful consideration of different ethical viewpoints.
- Bias in Algorithms: The algorithms used in autonomous vehicles can potentially reflect existing societal biases. Addressing bias in these systems is crucial to ensure fair and equitable outcomes.
Comparison of Safety Features, Regulations, and Potential Risks Across Levels
Level | Safety Features | Regulations | Potential Risks |
---|---|---|---|
Level 0 | Basic safety features (airbags, brakes) | Existing traffic laws and regulations | Human error, lack of vehicle safety systems |
Level 1 | Driver assistance systems | Existing traffic laws and regulations, emerging standards | Driver distraction, system malfunctions |
Level 2 | Partial automation, driver monitoring | Evolving standards, guidelines for automated functions | Inability to handle complex situations, driver complacency |
Level 3 | Conditional automation, automated intervention | Specific regulations for conditional automation | Transition failures, limited human oversight |
Level 4 | High automation, extensive sensors | Detailed regulations for autonomous operations | System failures, limited public acceptance |
Level 5 | Full automation | Comprehensive regulations for fully autonomous vehicles | Cybersecurity threats, algorithmic limitations |
Infrastructure Requirements
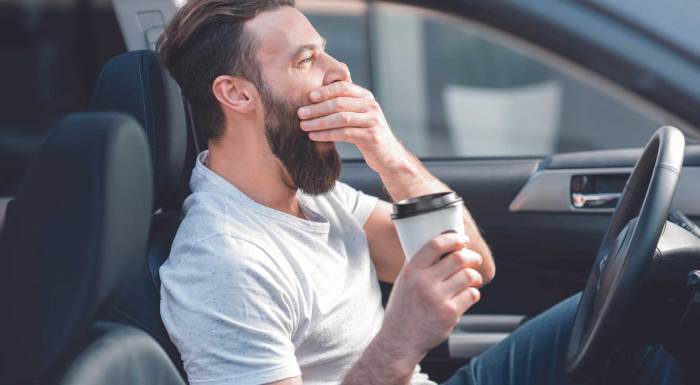
The infrastructure supporting autonomous vehicles plays a critical role in their safe and efficient operation. This involves a complex interplay of physical road elements, communication networks, and public policy frameworks. Proper infrastructure is essential to facilitate the transition to a fully autonomous future, ensuring seamless integration with existing traffic systems and promoting widespread adoption.
Infrastructure Needs for Different Levels of Autonomy
Different levels of autonomous driving require varying degrees of infrastructure support. Basic infrastructure, such as clear road markings and well-maintained roadways, is essential for all levels. However, as the level of autonomy increases, more sophisticated infrastructure, such as dedicated communication networks and sensor deployments, become increasingly crucial.
Road Markings and Signage
Clear and consistent road markings and signage are fundamental to autonomous vehicle operation. These markings provide vital information about lane boundaries, traffic flow patterns, and potential hazards. Advanced autonomous systems rely on precise and reliable interpretation of these markings for navigation and decision-making. The need for standardized and highly visible markings is crucial, particularly for intersections and complex junctions.
Communication Networks
Reliable communication networks are essential for autonomous vehicles to exchange data and interact with each other and infrastructure elements. These networks enable vehicles to communicate their intentions, positions, and potential hazards to surrounding vehicles. 5G and future wireless communication technologies offer enhanced capabilities for real-time data exchange and improved safety. Challenges in network coverage and reliability are a significant consideration, especially in rural areas or areas with poor infrastructure.
Infrastructure Integration Challenges and Solutions
Integrating autonomous vehicles into existing traffic systems presents several challenges. One significant concern is the compatibility of autonomous systems with existing infrastructure, requiring adaptations to existing traffic flow and signal patterns. Solutions include deploying adaptable traffic signals and dynamic traffic management systems that anticipate and react to autonomous vehicle movements. Another key challenge is the lack of standardized infrastructure, requiring a coordinated approach to infrastructure development and implementation across different regions.
Public Policy Role in Infrastructure Development
Public policy plays a pivotal role in shaping the infrastructure for autonomous vehicles. Government regulations and incentives can encourage the development and deployment of necessary infrastructure, while standardization efforts can improve compatibility across different autonomous vehicle systems. Investment in research and development for autonomous vehicle infrastructure is essential for long-term success. This includes funding for projects that test and evaluate new infrastructure technologies in real-world environments.
Table: Infrastructure Needs for Autonomous Driving Levels
Autonomous Driving Level | Infrastructure Needs | Potential Solutions | Challenges |
---|---|---|---|
Level 1 (Driver Assistance) | Clear road markings, lane delineation, basic signage | Improved maintenance of existing road infrastructure, clear signage, and advanced sensor deployment | Limited integration with existing traffic systems |
Level 2 (Partial Automation) | Advanced lane markings, adaptive traffic signals, limited communication infrastructure | Deploying adaptable traffic signals, advanced sensor deployment | Limited communication capabilities, inconsistent infrastructure |
Level 3 (Conditional Automation) | Dedicated communication networks, enhanced sensor networks, adaptive traffic management systems | 5G network infrastructure, dedicated communication channels for vehicles, intelligent traffic management systems | High initial cost of infrastructure upgrades, need for widespread adoption |
Level 4 (High Automation) | Advanced sensor networks, sophisticated communication systems, dedicated infrastructure areas | Advanced sensor deployments, autonomous traffic control systems, dedicated infrastructure development | Extensive infrastructure development costs, ensuring safety in highly automated environments |
Level 5 (Full Automation) | Fully integrated communication networks, advanced sensor networks, dedicated infrastructure | Developing fully autonomous traffic management systems, seamless integration with existing traffic patterns | High cost of infrastructure overhaul, complete reliance on technology |
Public Perception and Acceptance
Public perception plays a crucial role in the widespread adoption of autonomous vehicles. Understanding public sentiment, concerns, and expectations is essential for the successful integration of this technology into society. This understanding informs strategies to build trust and foster acceptance, ultimately accelerating the development and implementation of autonomous driving systems.Public acceptance of autonomous vehicles is influenced by a variety of factors, ranging from safety concerns and technological uncertainties to ethical considerations and economic implications.
Addressing these concerns proactively is vital for creating a positive and supportive environment that encourages the development and widespread deployment of this transformative technology.
Public Understanding and Perception of Autonomous Driving
Public understanding of autonomous driving varies significantly. While some grasp the basic concepts and potential benefits, others harbor misconceptions or lack a clear understanding of the technology. This disparity in understanding can lead to varying levels of acceptance and trust in autonomous vehicles.
Public Concerns and Anxieties about Autonomous Vehicles
Safety concerns are often paramount in public perception of autonomous vehicles. Questions about the reliability of the technology in various situations, particularly during unexpected events or malfunctions, contribute to anxieties. Furthermore, concerns about potential job displacement in the transportation sector, as well as ethical dilemmas surrounding decision-making in accident scenarios, further influence public opinion. The fear of losing control and the lack of human intervention in critical situations are prominent factors in this regard.
Data on Public Acceptance of Autonomous Driving
Data on public acceptance of autonomous vehicles reveals regional variations. Surveys and studies conducted in various countries and regions have shown different levels of acceptance, often influenced by local regulations, technological infrastructure, and cultural factors. For example, some regions might exhibit higher levels of acceptance due to strong government support or extensive public awareness campaigns. Conversely, regions with limited awareness or regulatory frameworks might show lower levels of acceptance.
Factors Influencing Public Perception
Several factors shape public perception of autonomous vehicles. These include, but are not limited to:
- Media Representation: The portrayal of autonomous vehicles in the media, whether positive or negative, significantly impacts public opinion. Sensationalized or overly dramatic portrayals can create apprehension, while positive and informative coverage can build trust.
- Safety Records: Early safety records of autonomous vehicles are critical. Demonstrating a consistent record of safe operation and minimizing accidents are essential to build public trust. A transparent approach to reporting incidents and lessons learned will also contribute to a positive perception.
- Government Regulations: Clear and comprehensive regulations governing autonomous vehicles can alleviate public concerns and foster a sense of security. Well-defined guidelines and protocols for testing, deployment, and eventual integration into the transportation system are important.
- Economic Impact: The perceived impact of autonomous vehicles on the job market and the economy can significantly influence public opinion. Strategies to mitigate potential job displacement and highlight new economic opportunities can help alleviate concerns.
- Personal Experiences: Direct or indirect personal experiences with autonomous vehicles or related technologies, such as ride-sharing services, can influence individual opinions. Providing opportunities for hands-on interaction or demonstrations can help dispel misconceptions and build trust.
Strategies for Fostering Public Acceptance
Strategies for fostering public acceptance of autonomous vehicles include:
- Transparent Communication: Open and transparent communication about the technology, its benefits, limitations, and safety measures is crucial. Engaging with the public through various channels, including educational materials and interactive demonstrations, is vital.
- Public Awareness Campaigns: Well-planned public awareness campaigns can effectively address concerns and educate the public about the technology. Clear and accessible information about autonomous driving, safety features, and potential societal impacts can help build public confidence.
- Collaboration with Stakeholders: Engaging with diverse stakeholders, including policymakers, industry representatives, and the public, can foster trust and address concerns collectively. Building consensus through collaboration can promote the responsible development and implementation of autonomous vehicles.
- Demonstrating Safety and Reliability: Extensive testing and validation are essential to demonstrate the safety and reliability of autonomous vehicles. Public demonstrations and showcasing real-world applications can effectively address concerns about safety and trust.
Economic Impacts and Job Displacement
Autonomous vehicles promise a revolution in transportation, but this shift will inevitably bring both opportunities and challenges to the global economy. The potential for increased efficiency and reduced costs in transportation is substantial, but the transition also necessitates careful consideration of the impact on various industries and the workforce. This section explores the complex economic implications of autonomous driving, including the potential for job displacement and the overall economic benefits and drawbacks.
Potential Economic Benefits
The adoption of autonomous vehicles has the potential to generate significant economic benefits across various sectors. Improved logistics and delivery systems, for example, can lead to substantial cost savings for businesses. Reduced traffic congestion and associated delays can boost productivity and efficiency for individuals and companies alike. Increased accessibility for people with disabilities is another potential benefit, improving quality of life and opening up new economic opportunities.
The creation of new industries focused on autonomous vehicle technology, such as software development and maintenance, could generate significant job growth in the future.
Potential Economic Drawbacks
While the potential benefits are significant, the transition to autonomous driving also presents economic challenges. The initial investment required for infrastructure upgrades and the development of autonomous vehicles can be substantial. Job displacement in traditional transportation sectors, such as trucking and taxi services, is a significant concern that needs careful management. The need for retraining and upskilling the workforce to adapt to the changing job market will also incur costs.
Furthermore, the potential for misuse of autonomous vehicles, such as in criminal activities, needs to be carefully addressed from an economic standpoint.
Job Displacement in Traditional Transportation Sectors
The introduction of autonomous vehicles is expected to significantly impact existing transportation industries. Truck drivers, taxi drivers, and ride-sharing service providers face potential job displacement as autonomous vehicles take over these roles. However, new job opportunities may arise in the areas of vehicle maintenance, software development, and data analysis. The transition period will require comprehensive retraining and reskilling programs to help workers adapt to the changing job market.
Autonomous driving levels are rapidly evolving, with advancements impacting various sectors. The integration of AI, particularly in the burgeoning field of Future of AI in business , is poised to revolutionize how we approach tasks like logistics and transportation. This ultimately translates to a more efficient and potentially safer future for autonomous driving systems.
The potential for job displacement requires careful planning and proactive measures to ensure a smooth transition for affected workers.
Economic Impact on Various Industries
The adoption of autonomous vehicles will ripple through various industries beyond transportation. The automotive industry will undergo a transformation, with a shift towards software-based technologies. Insurance companies will need to adapt their models to reflect the lower accident rates associated with autonomous vehicles. This transition could lead to significant changes in existing business models, and new business opportunities may emerge.
The need for new infrastructure, such as charging stations and autonomous vehicle maintenance facilities, will also stimulate economic activity.
Economic Implications in Different Regions
The economic implications of autonomous driving will vary significantly across different regions. Developed countries with strong technological infrastructure and a skilled workforce are likely to benefit more from the initial phase of the adoption of autonomous vehicles. Developing countries, on the other hand, may face challenges in terms of infrastructure development and workforce adaptation. The uneven distribution of economic benefits and burdens could lead to regional disparities and social tensions if not addressed properly.
Potential Economic Benefits, Drawbacks, and Impacted Sectors
Economic Aspect | Potential Benefits | Potential Drawbacks | Impacted Sectors |
---|---|---|---|
Transportation | Reduced congestion, improved efficiency, cost savings | Job displacement in trucking, taxi, and ride-sharing services | Trucking, taxi services, ride-sharing, logistics |
Automotive Industry | Shift to software-based technologies, new job creation | High initial investment in infrastructure | Automotive manufacturing, software development |
Insurance | Lower accident rates, new insurance models | Increased liability concerns, need for adaptation | Insurance companies |
Infrastructure | Stimulation of new infrastructure development | High upfront costs for infrastructure upgrades | Construction, energy |
Legal and Liability Issues
Autonomous vehicles present a complex web of legal and liability challenges. Establishing clear frameworks for responsibility in accidents involving these vehicles is crucial to fostering public trust and ensuring the safe integration of this technology. This necessitates a nuanced approach that considers the various actors involved, the level of automation, and the specific circumstances of each incident.Current legal frameworks, largely designed for traditional vehicles, are proving inadequate for the complexities of autonomous driving.
The shifting roles of human operators and automated systems require significant adaptation and clarification of existing laws. This evolution is not only a technical challenge but also a socio-legal one, demanding careful consideration of ethical principles and societal impact.
Current Legal Frameworks Regarding Liability
Existing legal frameworks for vehicle accidents typically place responsibility on the driver. However, autonomous vehicles introduce novel scenarios where determining liability becomes more intricate. The current legal landscape varies considerably across jurisdictions, posing significant challenges for uniform application and enforcement.
- In many jurisdictions, the current legal framework for accidents involving human-driven vehicles often serves as a baseline for determining responsibility in autonomous vehicle accidents. This is often done through a combination of negligence and strict liability principles.
- Liability in cases of accidents involving autonomous vehicles is often dependent on factors such as the level of automation, the nature of the accident, and the specific role of the human operator (if any) in the event. This often necessitates a nuanced approach that considers all aspects of the incident.
- Determining the extent of liability when a driver intervenes in a situation where an automated system could have reacted differently is a complex legal challenge. This requires careful consideration of the driver’s actions and the system’s design.
Challenges in Assigning Responsibility
Accidents involving autonomous vehicles present significant challenges in assigning responsibility. The complex interplay between human operators, automated systems, and environmental factors necessitates a multifaceted approach to legal analysis.
- The interplay of human and automated systems in an accident is a key factor in determining responsibility. Establishing clear lines of responsibility when the automated system malfunctions or when human intervention occurs is a crucial aspect of legal frameworks for autonomous driving.
- The question of responsibility shifts when a malfunction in the autonomous system leads to an accident. Determining whether the manufacturer, the vehicle owner, or the operator is liable in such a scenario is a significant legal concern. This often necessitates a thorough examination of the design and implementation of the autonomous system.
- Accidents caused by external factors, such as unforeseen environmental conditions or malicious hacking, introduce additional complexities in assigning responsibility. This highlights the need for adaptable legal frameworks capable of accommodating such novel scenarios.
Legal and Regulatory Landscape Across Regions
The legal and regulatory landscape for autonomous vehicles varies considerably across different regions. This disparity complicates the development and deployment of autonomous vehicles on a global scale.
- Different countries and regions have varying approaches to regulating autonomous vehicles. This diversity in regulations presents challenges in terms of standardization and consistency in the application of legal frameworks for autonomous driving.
- Some regions have developed specific regulations for autonomous vehicles, while others are still in the process of adapting existing laws. This creates an uneven playing field for companies developing and deploying autonomous vehicle technologies.
- The evolving nature of autonomous vehicle technology necessitates a dynamic approach to regulations. Legal frameworks must be flexible enough to accommodate advancements in technology without sacrificing safety standards.
Examples of Legal Cases or Precedents
While no widely recognized legal precedents directly address autonomous vehicle accidents yet, several legal cases provide valuable insights for shaping future frameworks. These cases often relate to issues of negligence, product liability, and the responsibility of manufacturers in vehicle accidents.
- Cases involving traditional vehicle accidents and their implications for the legal treatment of malfunctions in autonomous vehicle systems are important references for shaping future regulations and legal standards. These can include cases related to driver negligence, product defects, and the responsibilities of manufacturers.
Future Trends and Innovations: Levels Of Autonomous Driving
Autonomous driving is rapidly evolving, driven by continuous advancements in technology and research. The future promises significant enhancements in safety, efficiency, and accessibility, transforming the transportation landscape. These advancements are poised to redefine the very concept of mobility, impacting various aspects of daily life and the economy.
Emerging Technologies Enhancing Autonomous Driving
A multitude of emerging technologies are poised to significantly enhance autonomous driving systems. These advancements will not only improve the performance of current systems but also pave the way for entirely new capabilities. These innovations span a wide spectrum of fields, including sensor technology, machine learning, and communication systems.
Advancements in Sensor Technology
The accuracy and reliability of sensor data are crucial for safe and effective autonomous driving. Improvements in lidar, radar, and camera technology, along with the development of new sensor types, are constantly refining the perception capabilities of autonomous vehicles. This enhanced perception allows for more accurate object detection, improved situational awareness, and greater safety margins. For instance, advancements in lidar technology now allow for more precise 3D mapping, enabling autonomous vehicles to navigate complex environments more effectively.
Role of Machine Learning in Autonomous Driving
Machine learning algorithms play a critical role in enabling autonomous vehicles to learn from data and adapt to various driving conditions. The application of machine learning is fundamental to the development of more sophisticated decision-making capabilities in autonomous vehicles. Deep learning algorithms, for example, are being trained on vast datasets of driving scenarios to improve object recognition, predict potential hazards, and optimize driving strategies.
Impact of Advanced Communication Systems
Advanced communication systems are essential for enabling seamless and coordinated interactions between autonomous vehicles and the surrounding infrastructure. The use of 5G and other high-bandwidth communication technologies will allow for real-time data exchange between vehicles and infrastructure, enabling vehicles to share information on traffic conditions, potential hazards, and optimal routes. This communication capability allows for proactive responses to changing conditions, improving safety and efficiency.
Predicted Impact on Levels of Autonomy
The advancements in technology are anticipated to propel the evolution of autonomous driving levels. The combination of improved sensors, more sophisticated algorithms, and advanced communication systems will likely push the boundaries of what’s possible, leading to higher levels of autonomy and wider application in diverse environments. For example, the improved processing power and communication capabilities will enable Level 4 and Level 5 autonomous vehicles to navigate more complex and dynamic environments.
Furthermore, advancements in machine learning will empower autonomous vehicles to learn and adapt to novel situations and environmental changes, thereby increasing their operational efficiency and safety.
Societal Impacts
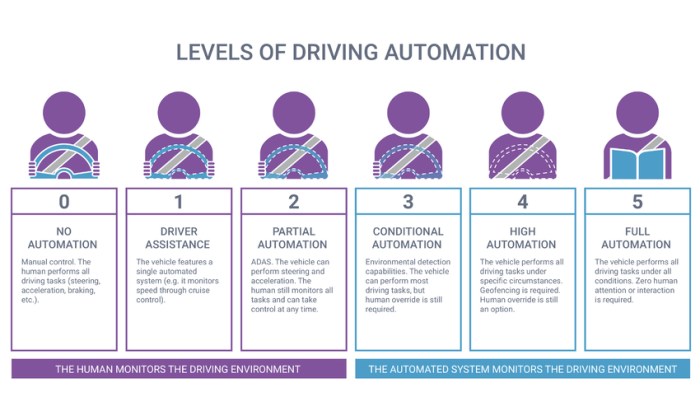
Autonomous driving promises a profound reshaping of society, impacting transportation, urban planning, and employment in significant ways. The transition to widespread adoption of autonomous vehicles will necessitate a careful consideration of both the potential benefits and drawbacks. This shift will require adaptation across numerous societal facets, from personal mobility to infrastructure design.
Transformation of Transportation Habits
The introduction of autonomous vehicles will fundamentally alter how people interact with transportation. Increased convenience and accessibility are anticipated, potentially leading to changes in commuting patterns and the use of personal vehicles. Autonomous ride-sharing services could drastically reduce the number of privately owned vehicles on the road, impacting parking requirements and urban traffic flow. This will also influence the design and development of public transportation systems.
Impact on Urban Planning
Autonomous vehicles will likely trigger adjustments in urban planning strategies. The potential for increased efficiency in traffic flow and reduced congestion will necessitate modifications to road networks, parking structures, and even the design of urban spaces. Flexible transportation options may lead to the reconfiguration of residential areas and commercial districts, fostering new possibilities for urban development. Areas currently designated for parking might be repurposed for pedestrian areas, green spaces, or other public amenities.
Impact on Employment
The widespread adoption of autonomous vehicles will undoubtedly impact various sectors of the workforce. Jobs directly related to driving, such as truck drivers, taxi drivers, and delivery drivers, may experience significant displacement. However, new opportunities will emerge in areas such as autonomous vehicle maintenance, software development, and data analysis. The transition will require substantial workforce retraining and upskilling programs to ensure a smooth adaptation to the changing job market.
Societal Benefits and Drawbacks
Autonomous driving holds several potential benefits for society. Enhanced safety due to reduced human error, increased efficiency in traffic flow, and greater accessibility for individuals with mobility limitations are prominent advantages. However, potential drawbacks include the potential for job displacement, the need for substantial infrastructure upgrades, and concerns about data security and privacy. The societal benefits and drawbacks must be carefully balanced to ensure a positive impact on society.
Table: Societal Impacts of Autonomous Driving
Domain | Potential Impacts |
---|---|
Transportation | Increased efficiency, reduced congestion, enhanced safety, greater accessibility for certain groups. Potential for decreased personal vehicle ownership, impact on public transportation systems. |
Urban Planning | Re-evaluation of road networks, parking requirements, and urban space design. Potential for more pedestrian-friendly and green urban environments. |
Employment | Displacement of jobs in driving-related professions, emergence of new job opportunities in maintenance, software development, and data analysis. Requirement for workforce retraining and upskilling. |
Safety | Reduced accident rates, improved response times for emergency services. Increased need for robust safety protocols and redundancy in autonomous systems. |
Accessibility | Improved mobility for people with disabilities or limited mobility. Potential for greater independence and freedom of movement. |
Case Studies of Autonomous Vehicle Deployment
Autonomous vehicle deployments are a crucial aspect of evaluating the viability and practical application of these technologies. Real-world implementations provide invaluable insights into the challenges and successes encountered, enabling a more nuanced understanding of the field’s current state and future potential. These case studies offer a glimpse into the practical application of various levels of autonomy and the factors influencing their success or failure.
Autonomous Vehicle Deployments in Specific Regions
Various regions have undertaken autonomous vehicle deployments, each presenting unique contexts and outcomes. These deployments are often tailored to specific local conditions, infrastructure, and regulations. Successes and failures highlight the complex interplay of technological, regulatory, and societal factors in shaping the adoption of autonomous vehicles.
- Pittsburgh, USA: The deployment of autonomous vehicles in Pittsburgh focused on testing and demonstration in a controlled environment. This involved a collaboration between various stakeholders, including academic institutions, tech companies, and local government. Key successes included valuable data collection for improving vehicle algorithms and public awareness campaigns. However, challenges included adapting to unpredictable weather conditions and ensuring public safety amidst changing traffic patterns.
This deployment primarily focused on Level 4 autonomy, demonstrating the capabilities of self-driving vehicles in a real-world setting.
- California, USA: California has been a significant testing ground for autonomous vehicle technologies. The state’s supportive regulatory environment and presence of major tech companies have spurred significant progress. Several companies have tested and deployed vehicles on public roads, often in specific areas with limited traffic and well-defined routes. Successes include advancements in sensor technology, navigation systems, and route optimization.
Challenges include adapting to complex and unpredictable driving scenarios, ensuring public safety, and overcoming regulatory hurdles. Deployment examples here include Level 3 and Level 4 autonomous vehicles in specific conditions, emphasizing the need for a careful and measured approach to integrating these technologies.
- Singapore: Singapore’s deployment of autonomous vehicles focuses on integrating them into existing infrastructure. Their efforts emphasize developing and testing autonomous vehicles in controlled environments, such as specific roads and highways. Successes include successful collaborations with technology companies and infrastructure partners. Challenges include the integration of autonomous vehicles into existing traffic management systems and addressing the potential impact on public transportation.
This deployment showcases the practical application of autonomous vehicle technology in a densely populated urban environment and focuses on the need for integrated infrastructure support.
Challenges and Success Factors in Autonomous Vehicle Deployments
The successful deployment of autonomous vehicles relies on a complex interplay of technological, regulatory, and societal factors. These factors significantly influence the outcomes of these deployments, shaping the experience and acceptability of this technology.
- Technological Challenges: Developing robust and reliable autonomous driving systems is crucial. Challenges include overcoming unpredictable weather conditions, handling complex and unexpected road scenarios, and ensuring the reliability of sensors and algorithms in various environmental conditions. Successes include improvements in sensor accuracy and data processing, enabling vehicles to navigate diverse and dynamic environments. These advancements often reflect the progression from Level 2 to Level 4 autonomous systems.
Autonomous driving levels are progressing rapidly, from basic driver assistance to fully hands-off operation. Securing your digital assets, especially cryptocurrencies, is paramount in this evolving landscape. Using a robust crypto wallet like the ones listed at Best crypto wallets is crucial for safeguarding your investments, no matter the level of autonomous driving technology you’re utilizing. This ensures peace of mind while experiencing the future of transportation.
- Regulatory Hurdles: Establishing clear and comprehensive regulations for autonomous vehicles is essential. Challenges include defining liability in case of accidents, addressing the legal and ethical implications of autonomous decision-making, and ensuring compliance with existing traffic laws. Successes include the development of specific regulations for autonomous vehicles, allowing for the safe integration of these technologies into the existing traffic system.
Such regulations often reflect the evolving levels of autonomy being deployed.
- Public Acceptance and Perception: Public perception and acceptance of autonomous vehicles are vital for their widespread adoption. Challenges include overcoming concerns about safety, job displacement, and the overall impact on society. Successes include public awareness campaigns, community engagement programs, and clear communication strategies to address concerns and foster trust. Public acceptance is often a critical factor for successful Level 5 deployments.
Summary Table of Case Studies
Location | Deployment Focus | Key Outcomes |
---|---|---|
Pittsburgh, USA | Level 4 testing and demonstration | Data collection, public awareness, algorithm improvements |
California, USA | Level 3 and 4 testing on public roads | Sensor technology advancements, route optimization, regulatory progress |
Singapore | Integration into existing infrastructure | Collaboration with tech companies, infrastructure partners, traffic management system integration |
Autonomous Driving and the Environment
Autonomous vehicles hold the potential to reshape transportation, but their environmental impact is a crucial consideration. While promising advancements in efficiency and safety are evident, the full environmental footprint of these vehicles is multifaceted and depends heavily on various factors. The design, energy sources, and operational strategies all play a vital role in determining their impact on the planet.The transition to autonomous driving presents a complex interplay between technological advancements and environmental sustainability.
This includes exploring the energy sources used, the materials employed in vehicle construction, and the potential for reduced traffic congestion and emissions. Analyzing these factors is essential for developing a sustainable and responsible autonomous vehicle ecosystem.
Environmental Impact of Autonomous Vehicles
The environmental impact of autonomous vehicles is a multifaceted issue, encompassing design choices, energy consumption, and the potential for reduced congestion and emissions. Understanding these interconnected factors is crucial for evaluating the overall impact of this emerging technology.
Potential for Reduced Traffic Congestion and Emissions
Autonomous vehicles, with their ability to communicate and coordinate movements, show promise for optimizing traffic flow and reducing congestion. This optimization can translate into lower fuel consumption and reduced emissions compared to traditional vehicles operating in congested traffic. Improved traffic flow can potentially decrease the time vehicles spend idling, further minimizing emissions. Pilot programs and simulations are already demonstrating the potential for reduced congestion and emissions.
Sustainable Practices in Autonomous Vehicle Design
Sustainable design principles are increasingly important in the development of autonomous vehicles. This includes the use of recycled materials in construction, the adoption of lightweight design to reduce energy consumption, and the integration of renewable energy sources for vehicle operation. Examples include utilizing advanced battery technologies, exploring hydrogen fuel cell options, and optimizing aerodynamic designs for better fuel efficiency.
Companies are actively researching and implementing these sustainable practices to mitigate the environmental footprint of autonomous vehicles.
Energy Consumption and Carbon Footprint of Different Levels of Autonomous Driving
The energy consumption and carbon footprint of autonomous vehicles vary significantly depending on the level of autonomy. Level 0 (no automation) vehicles consume energy based on the driver’s habits and the vehicle’s efficiency. Higher levels of automation, while potentially reducing driver fatigue and improving efficiency, could still differ in their energy consumption depending on factors such as the specific technology used, infrastructure support, and driving patterns.
For example, Level 5 vehicles, operating fully autonomously, may exhibit a slightly higher energy consumption due to the use of advanced sensors and communication systems, yet could potentially offset this by optimizing traffic flow and reducing idling time.
Comparison of Environmental Impact
Feature | Autonomous Vehicles (Potential) | Traditional Vehicles |
---|---|---|
Fuel Efficiency | Potentially higher due to optimized routing and traffic management | Varying, depending on vehicle type and driving habits |
Emissions | Potentially lower due to reduced congestion and optimized routes | Varying, depending on vehicle type and driving habits |
Congestion | Potentially reduced through optimized traffic flow | High, leading to increased fuel consumption and emissions |
Safety | Potentially improved through enhanced driving capabilities | Varying, depending on driver skill and experience |
Summary
In conclusion, Levels of autonomous driving are a complex interplay of technology, safety, and societal impact. The journey toward widespread adoption involves overcoming technological hurdles, addressing safety concerns, and fostering public acceptance. As the technology matures, we anticipate significant changes in transportation and daily life.
FAQ Insights
What are the key differences between Level 2 and Level 3 autonomous driving?
Level 2 autonomous driving systems offer driver assistance features, but the driver remains ultimately responsible. Level 3 systems, on the other hand, can take over control of the vehicle under certain conditions, but the driver needs to be prepared to take back control immediately. The key difference is the degree of human intervention required.
What are the most common public concerns about autonomous vehicles?
Public concerns often center around safety, liability in accidents, and the potential for job displacement. There are also questions about data privacy and the ethical implications of making decisions on behalf of human drivers.
How do infrastructure needs vary for different levels of autonomous driving?
The infrastructure required for higher levels of autonomy necessitates more sophisticated communication networks, dedicated lanes, and advanced road markings, all designed to support the safe operation of vehicles without human intervention.
What are the potential environmental impacts of autonomous driving?
Autonomous vehicles can potentially reduce congestion and emissions through optimized traffic flow. However, the energy consumption and carbon footprint of the vehicles themselves, and the production of the required technology, are also factors to consider.